Effective Ways to Interpret P-Value for Current Data in 2025
As we delve into the world of data analysis and scientific research, understanding p-values is essential for interpreting statistical significance and making informed decisions. In 2025, as data continues to grow exponentially, the ability to accurately interpret p-values is more critical than ever. The p-value, a cornerstone of hypothesis testing, helps researchers gauge the strength of evidence against the null hypothesis, which posits that no effect or difference exists. By the end of this article, you will grasp not only the definition and interpretation of p-values but also their significance in research methodologies and empirical studies. We will explore its limitations, the relationship between p-values and effect sizes, and the implications of misinterpretation, providing you with a holistic view of how this statistical measure plays a pivotal role in data-driven decisions.
The key takeaways include a comprehensive understanding of the p-value definition and meaning, the importance of significance levels, and how to interpret results in the context of statistical inference. By linking p-values to research findings and data significance, you will be empowered to apply these concepts effectively in your future analyses.
Understanding P-Value and Its Importance
Defining P-Value in Hypothesis Testing
The p-value, in its essence, represents the probability of observing test results at least as extreme as the results actually observed, given that the null hypothesis is true. Essentially, it quantifies the evidence against the null hypothesis in any statistical test. When conducting hypothesis testing, researchers set a significance level, commonly denoted as alpha (α), which indicates the threshold at which results are deemed statistically significant. This level is often set at 0.05, meaning that there's a 5% risk of concluding that a difference exists when there is no actual difference.
The Role of Statistical Significance
Statistical significance informs researchers whether their findings are likely due to chance. A smaller p-value indicates stronger evidence against the null hypothesis, while a p-value greater than the significance level suggests that the observed effect is likely trivial or non-existent. This critical threshold helps in making decisions about the necessity of further research or the implementation of findings. Notably, researchers must also consider the context of their study, including sample size and data variability, as these can significantly influence p-values and their interpretations.
P-Value Thresholds and Significance Levels
Different fields may employ various thresholds for p-values to declare statistical significance. In clinical settings, a more stringent threshold of 0.01 could be favored to minimize Type I errors — the erroneous rejection of the null hypothesis. Conversely, a study with larger sample sizes may detect smaller effects, thereby influencing the practicality of p-value thresholds. Understanding the balance between significance levels and real-world relevance becomes crucial in research, influencing how results are presented and interpreted by peers and in publications.
Calculating and Interpreting P-Values
Methods to Calculate P-Values
P-values can be calculated using various statistical tests, including t-tests, chi-square tests, ANOVA, and regression analyses, among others. These statistical tools serve as frameworks for deriving p-values. For instance, in a t-test, the p-value informs the researcher whether the means of two groups are significantly different. When running such tests, statistical software often automates calculations, but understanding the underlying methodology remains essential for proper interpretation.
Examples of P-Value Interpretation
Let’s consider a practical example — a study examining the efficacy of a new drug compared to a placebo. A p-value of 0.03 would suggest that there is a statistically significant difference in efficacy at the 0.05 significance level, implying that only a 3% probability exists that the observed difference is due to chance. In contrast, a p-value of 0.08 would lead researchers to accept the null hypothesis, believing that there isn’t sufficient evidence to support the drug's effectiveness. These examples illustrate how crucial the p-value is in validating hypotheses and directing further research.
P-Value Limitations and Misinterpretations
Despite its widespread use, the p-value is often criticized for its limitations. Researchers may misinterpret a p-value as the probability that the null hypothesis is true or as an absolute measure of effect size. This misunderstanding can lead to flawed conclusions and decisions in the research process. Furthermore, solely relying on p-values without considering confidence intervals or effect sizes can result in incomplete assessments of research findings. Therefore, robust statistical reasoning is critical for accurate interpretation and utilization of p-values.
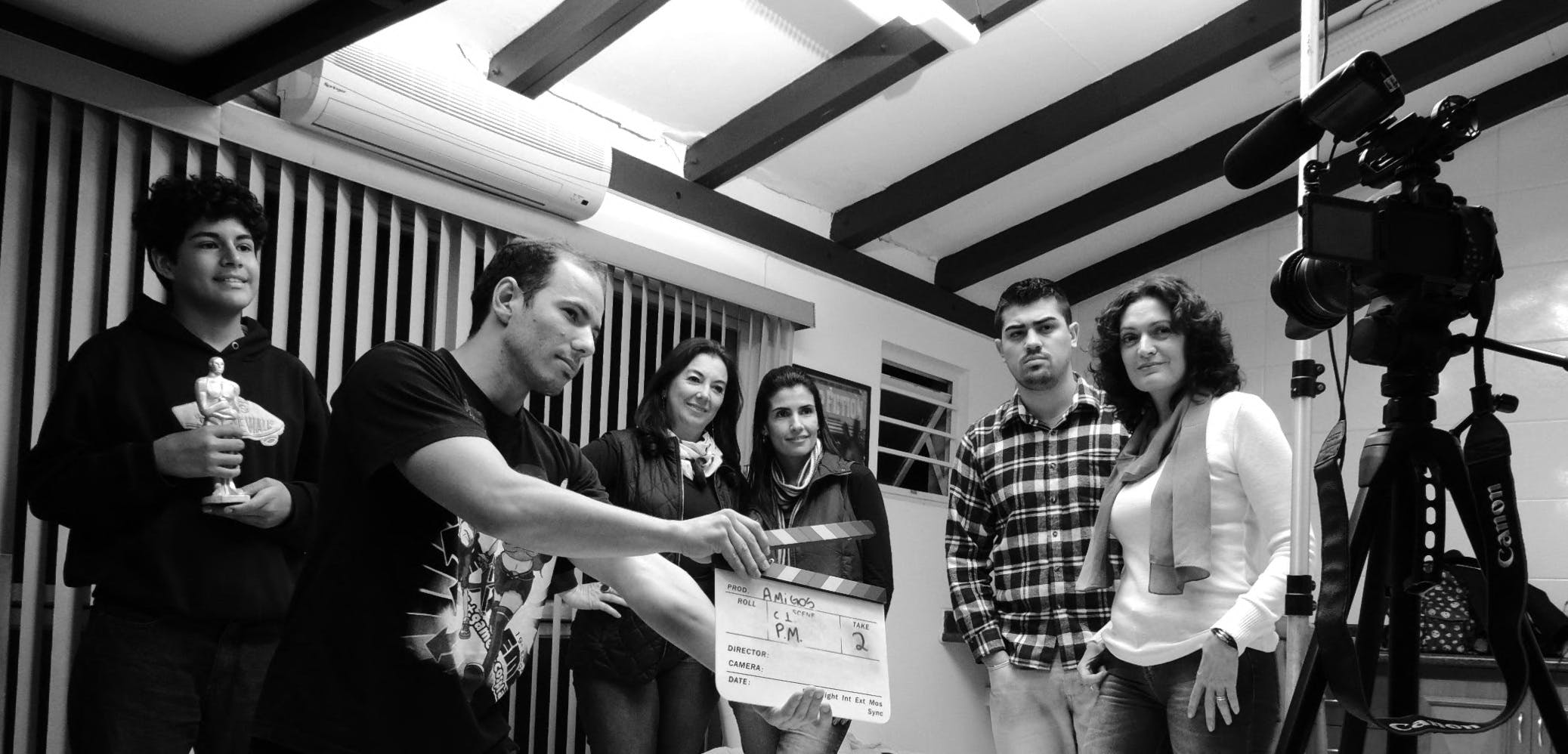
Applying P-Values in Scientific Research
The Relationship Between P-Values and Effect Size
The concept of effect size provides a different lens through which researchers can evaluate their findings beyond mere statistical significance. While a p-value indicates whether an effect exists, the effect size quantifies how strong that effect is. For example, in psychological studies, a small p-value might signal a statistically significant finding, but without a meaningful effect size, the practical implications of that finding could be minimal. It is vital for researchers to report both p-values and effect sizes to convey the full context of their results.
Understanding P-Values in Inferential Statistics
In inferential statistics, p-values play a vital role in evaluating hypotheses based on sample data and making generalized conclusions about populations. Researchers utilize p-values from specific samples to infer about broader trends. This reasoning extends to various areas including clinical trials, market research, and social sciences, where the ability to draw broad conclusions from finite data is paramount. The interpretation of p-values must therefore be communicated transparently to ensure accurate understanding in practical applications.
P-Value Reporting Guidelines and Ethical Considerations
With transparency in scientific research becoming ever more critical, strict guidelines have emerged around the reporting of p-values. Researchers are encouraged to share exact p-values and avoid arbitrary cutoffs while presenting their findings. This practice not only enhances the credibility of results but also addresses concerns regarding publication bias and the replication crisis within the scientific community. By fostering enhanced transparency and adherence to ethical standards, the integrity of research findings improves significantly.
Alternatives and Extending the Use of P-Values
Alternative Approaches to P-Values
In recent years, alternatives to p-values have garnered attention in the statistical community. Bayesian statistics, for example, allows researchers to incorporate prior knowledge and provides a more nuanced interpretation of results. This framework helps quantify uncertainty in a manner that traditional p-value approaches may not. As the field progresses, integrating approaches like Bayesian methods with traditional significance testing could lead to more robust conclusions in scientific inquiry.
Importance of Statistical Literacy in Interpreting P-Values
As the complexity of data analysis continues to grow, enhancing statistical literacy among researchers, practitioners, and policy-makers is paramount. This ensures the accurate interpretation of p-values and promotes sound decision-making in research contexts. Educational initiatives and workshops providing insights into statistical reasoning can help mitigate common misconceptions surrounding p-values, thereby fostering higher quality scientific research and data interpretation for practical applications.
Case Studies and Empirical Research Using P-Values
To illustrate the practical applications of p-values in research, several case studies can provide insightful perspectives. For instance, clinical trials evaluating new therapies frequently employ p-values to determine efficacy and safety, influencing treatment protocols worldwide. Similarly, studies in social sciences assess correlations through p-values, revealing significant relationships that shape government policies and community interventions. These empirical examples underscore the relevance and importance of p-values in demonstrating research validity.
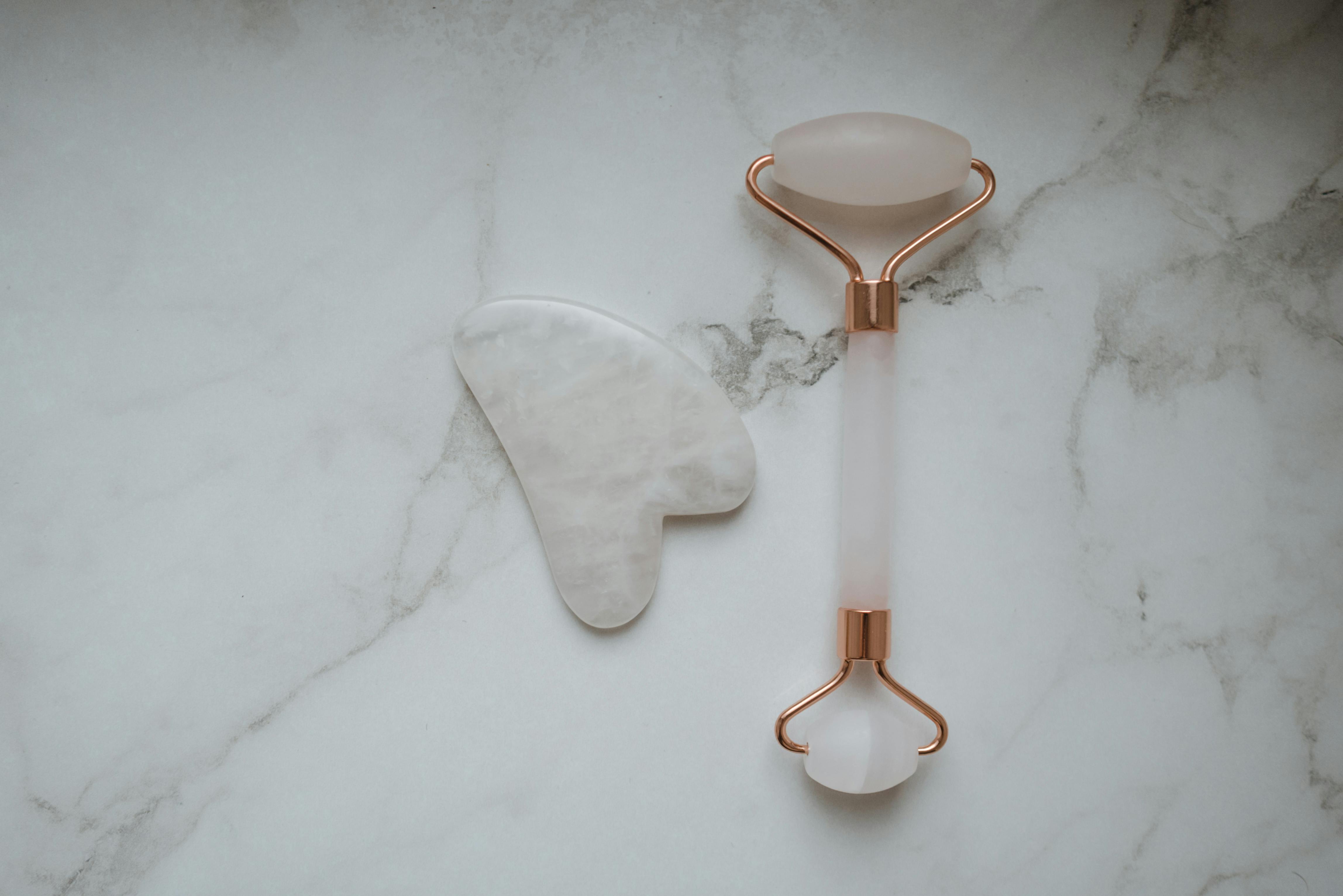
Conclusion: The Future of P-Value Interpretation
As we look forward to 2025 and beyond, interpretations of p-values will continue to evolve along with advancements in statistical methods and research practices. Embracing alternatives to p-values while improving statistical literacy will be pivotal in enhancing the quality of scientific discourse. Researchers must adopt a holistic view of p-values within their context, respecting both the limitations and strengths of this statistical measure. By doing so, they will contribute to a more robust understanding of data analysis and foster an evidence-based approach to scientific research.